case study
Projecting Growth Using Predictive Data Science
How a major subscription-based organization gained from Nómadas' experience in forecasting subscribers, churn, user lifetime value, and revenue.
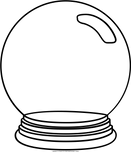
about the client
This large subscription business had already amassed 2.5 million loyal monthly subscribers and was regularly spending millions in marketing to continue to gain additional users. They had taken a stab at modeling manually and were ready to take things up a level.
overview
Our client's goal was to proactively use data to make and validate decisions. We transitioned them to a mature approach using the powers of predictive modeling. We were able to quantify, in advance, their return on investment for media spend, the impact of pricing model changes, and the impact of product design improvements on key metrics.
For those unfamiliar with predictive analytics, it involves taking past data and other factors to create a combination of mathematical equations, called a model. This model generates a forecast of what one or many other data points key to success will be at a specific point in the future. Given the complexity of the data and the many factors often used to achieve an accurate prediction, a programming language is used to simplify and speed up the time it takes to run and maintain a model.
Naturally, we simulated our prediction in a historical setting to validate it against past actuals; the model maintains a consistent and trustworthy industry accepted variance of under 10% margin of error on a daily basis.
Beyond simply creating work and tossing it over the fence, our goal was to educate and enable internal resources to not be dependent on external support. We offered follow-up services such as automating data ingestion and execution by hosting the model in cloud (to save ongoing internal resources needed to run the model) and machine learning modeling to account for seasonality and user traits to allow for ongoing improvements in predictive accuracy.
approach
MATURE
Sometimes it is not best to not throw the baby out with the bath water. We wanted to mature and not replace. While there were many improvements on the existing approach, we were sure to fully evaluate the existing approach, retain business logic, and protect methodologies where it made sense to ensure continuity in our advanced model.
CLEAN
Data models, as with most things, you get out what you put in. We spent significant time learning and asking questions to fully understand all the data points. This is critical to ensure there are no assumptions or misunderstandings on how to use and weight various factors to create the foundation for clean data.
BESPOKE
Customization to various edge cases were critical to account for multiple types of user journeys such as various free trials, promotional calendars, pricing model changes, past product changes as well as the tricky accommodation of users reactivating their subscription.
EFFICIENT
The client's existing analysis was built within Microsoft Excel and Google Sheets and challenging to maintain, so our task was to upgrade to a programming language not only capable of gracefully handling all of the factors but a significantly more efficient use of time to run and modify. The model was created in Python using SQL to pull data from Snowflake data warehouse and GitHub to store the files for easy in-house use.
solutions
-
Client requirements gathering and goal setting
-
Investigate and clean existing data sets
-
Plot output schema
-
Create new model with low band and high band prediction range
-
Fine tune the model
-
Ongoing model maintenance validation against actuals
results
Months
Internal Time Saved*
<10%
Error Margin
$Millions
Marketing Spend Protected*
* additional visibility intentionally obscured for client confidentiality